Leveraging Zero-Knowledge Proofs with AI Practical Applications
Table of Contents
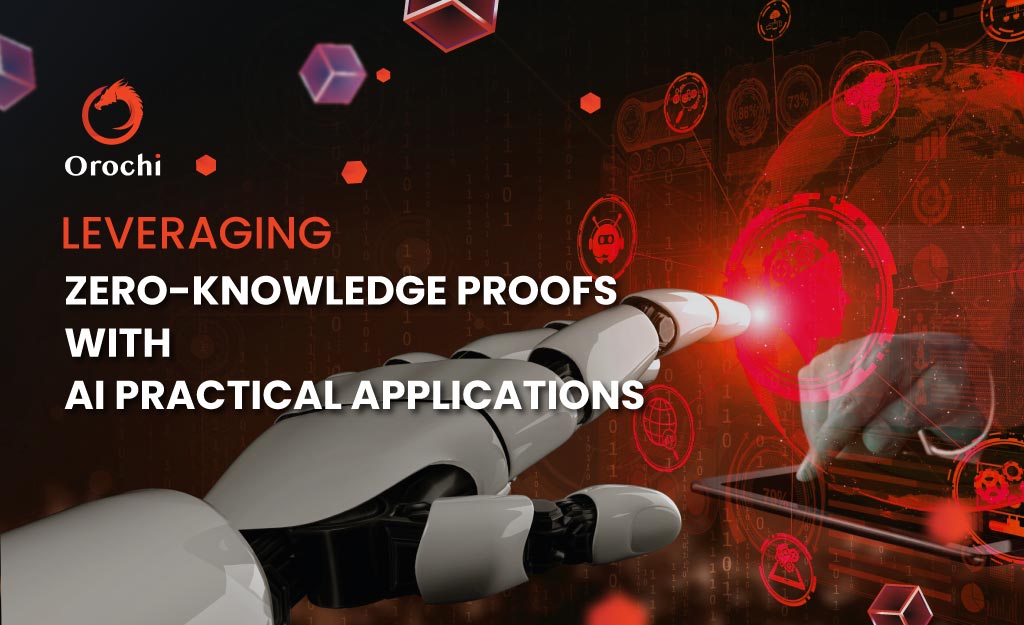
In machine learning, where vast amounts of data fuel the remarkable capabilities of AI models, a pressing concern emerges: the erosion of privacy as data volumes surge. The very foundation of machine learning rests upon the trust users place in these models to handle their data responsibly. However, as data privacy issues come to the forefront, the need for accountability and security becomes paramount. This article explores a groundbreaking solution — zero-knowledge proofs — that harmonizes the power of machine learning with the imperative of data privacy and integrity.
I. The Promise of Zero-Knowledge Proofs with AI Implementation
In the ever-evolving landscape of artificial intelligence, the integration of zero-knowledge proofs brings forth a revolutionary concept that holds the potential to reshape the fundamentals of machine learning. This cryptographic technique introduces an unprecedented layer of privacy and accountability, addressing the critical concern of data privacy and trustworthiness in AI implementation.
How Zero-Knowledge Proofs Enhance Privacy and Trust Implementation with AI?
Zero-knowledge proofs offer a solution that transcends the conventional trade-off between privacy and utility. In the realm of machine learning, where models learn from vast datasets to provide valuable insights and predictions, concerns regarding the misuse or exposure of sensitive data have been a persistent obstacle. Zero-knowledge proofs enable computations to be verified without disclosing the actual data involved in those computations. This means that machine learning models can operate on private data, generating accurate outcomes without revealing the underlying sensitive information. This innovation translates to increased trust between users and AI systems, as individuals can be assured that their personal data remains hidden while still contributing to valuable model outcomes.
The Balance Between Personalization and Security with AI?
Machine learning models have become integral to various applications, offering personalized experiences that cater to individual preferences. However, the pursuit of personalization has sometimes clashed with concerns about data security and misuse. Zero-knowledge proofs strike a harmonious balance between these two seemingly opposing aspects. As models learn from private data, users can enjoy the benefits of personalized recommendations, predictive insights, and tailored services without relinquishing control over their personal information. This balance reinforces the notion that AI can be a force for good while maintaining the highest standards of data privacy and security.
II. On-Chain Use Cases
1. Privacy-Preserving Credit Scores
Cryptocurrency markets have transcended geographical borders, introducing the challenge of assessing the creditworthiness of international borrowers who often operate pseudonymously. Employing a machine learning model to evaluate borrowers' trustworthiness, all while preserving their privacy, opens the door to a realm of possibilities. With zero-knowledge proofs, lenders can match borrowers with ideal counterparts, safeguarding both financial interests and data privacy.
2. Private Know-Your-Customer (KYC) Processes
Modern KYC processes demand users to divulge personal information for verification, raising privacy concerns. The fusion of machine learning and zero-knowledge proofs offers a novel approach. This solution can accurately conduct liveness tests and verify user identities without compromising their personal data. Through cryptographic proofs, the model attests to accurate execution while never exposing sensitive details.
3. Accurate Stablecoin Exchange Rates
Stablecoins rely on accurate exchange rate data, often sourced off-chain. The introduction of machine learning to refine this data, coupled with zero-knowledge proofs, elevates the accuracy and reliability of oracles. These cryptographic proofs validate the correct computation of exchange rates, fostering a trustable bridge between the blockchain and real-world data.
III. Off-Chain Use Cases
1. High-Assurance Industries
Industries like defense, autonomous vehicles, and medical diagnostics depend on the unadulterated precision of machine learning outcomes. The integration of zero-knowledge proofs becomes indispensable, verifying that sensor inputs are analyzed correctly. This is pivotal for applications where lives hang in the balance, guaranteeing results untarnished by malicious interventions.
2. Protecting Proprietary Machine Learning Models
Enterprises often develop proprietary machine learning models that must remain confidential. As the significance of these models grows, so does the need for proof of their origin. Zero-knowledge proofs come to the rescue, allowing companies to validate results without disclosing the intricate workings of their models, preserving proprietary insights while bolstering trust.
IV. Challenges and Considerations
The integration of zero-knowledge proofs into the fabric of AI implementation is not without challenges. While the benefits are evident, a comprehensive understanding of the obstacles is essential to ensure a seamless and effective deployment of this transformative technology.
Privacy Concerns in Modern Machine Learning
In today's data-driven world, the revelations of unauthorized data access, breaches, and privacy infringements have created an urgent need for enhanced data protection mechanisms. Users and organizations are rightfully concerned about the security of their information, and machine learning is no exception. Traditional machine learning models often necessitate access to raw data, increasing the risk of potential breaches. Zero-knowledge proofs emerge as a potent solution, addressing these privacy concerns by allowing computations to occur on encrypted data without exposing the raw information itself.
The Vulnerabilities of Traditional Data Sharing
Conventional data sharing practices come with inherent vulnerabilities. The exchange of raw data for model training or validation poses potential risks, as data could be intercepted or misused during transit. Moreover, once data is shared, control over its usage is compromised. Zero-knowledge proofs circumvent these vulnerabilities by enabling computations on encrypted data, eliminating the need to share raw data while still attesting to the accuracy of the results.
Technical Aspects of Incorporating Zero-Knowledge Proofs
The implementation of zero-knowledge proofs requires a sound understanding of cryptographic techniques and their integration into the machine learning pipeline. Developers need to navigate the intricacies of cryptographic protocols, ensuring that the proofs are generated and verified correctly. While the technology is promising, its successful incorporation demands careful planning and expertise in both machine learning and cryptography.
Ensuring Data Integrity and Accuracy
Maintaining the integrity and accuracy of computations is paramount in AI implementation. Zero-knowledge proofs offer a mechanism to validate the correctness of computations while keeping the underlying data confidential. However, ensuring that the proofs themselves are generated accurately and that the verification process is robust is a challenge that must be tackled to guarantee the reliability of the entire system.
Conclusion
As artificial intelligence permeates every facet of our existence, the assurance of trustworthy models and data gains paramount importance. Zero-knowledge proofs offer a gateway to a future where AI-powered insights coexist harmoniously with individual privacy. With platforms like Aleo simplifying the development of zero-knowledge algorithms, machine learning developers can focus on their core expertise: forging algorithms and models that illuminate our world with newfound wisdom.
About Orochi Network
Orochi Network is a cutting-edge zkOS (An operating system based on zero-knowledge proof) designed to tackle the challenges of computation limitation, data correctness, and data availability in the Web3 industry. With the well-rounded solutions for Web3 Applications, Orochi Network omits the current performance-related barriers and makes ways for more comprehensive dApps hence, becoming the backbone of Web3's infrastructure landscape.
Categories
Event Recap
3
Misc
56
Monthly Report
1
Oracles
4
Orand
3
Orosign
19
Partnership
20
Verifiable Random Function
9
Web3
86
Zero-Knowledge Proofs
36
Top Posts
1
Introducing Orochi Network - The Operating System For High Performance dApp And Metaverse
10 January 2023
2
Orosign Wallet 101: How to get started?
03 February 2023
3
Validity Proofs vs. Fraud Proofs: An Explanation
06 January 2023
4
Introducing Orosign Multisignature Wallet - A Self-Managing Mobile App For Digital Assets
06 January 2023
5
Introducing X-ORO Points: Opportunity to jump into Orochi Network's Token Whitelist
22 March 2024
6
Discovering the Orochi Retroactive Adventure: Origin, Oro Wild, and Oro Futuristic
21 March 2024
7
Introducing Orand: Your Trustless Source of Randomness
20 February 2023
8
Compete, Connect, Conquer: Orochi Network's Leaderboard Challenge Begins
01 April 2024
Tag
Orand
NFT
Misc
Web3
Partnership Announcement
Layer 2
Event Recap
Immutable Ledger
Oracles
Verifiable Random Function
Zero-Knowledge Proofs
Multisignature Wallet