Understand the Decentralized AI Landscape: A Friendly Exploration
Table of Contents
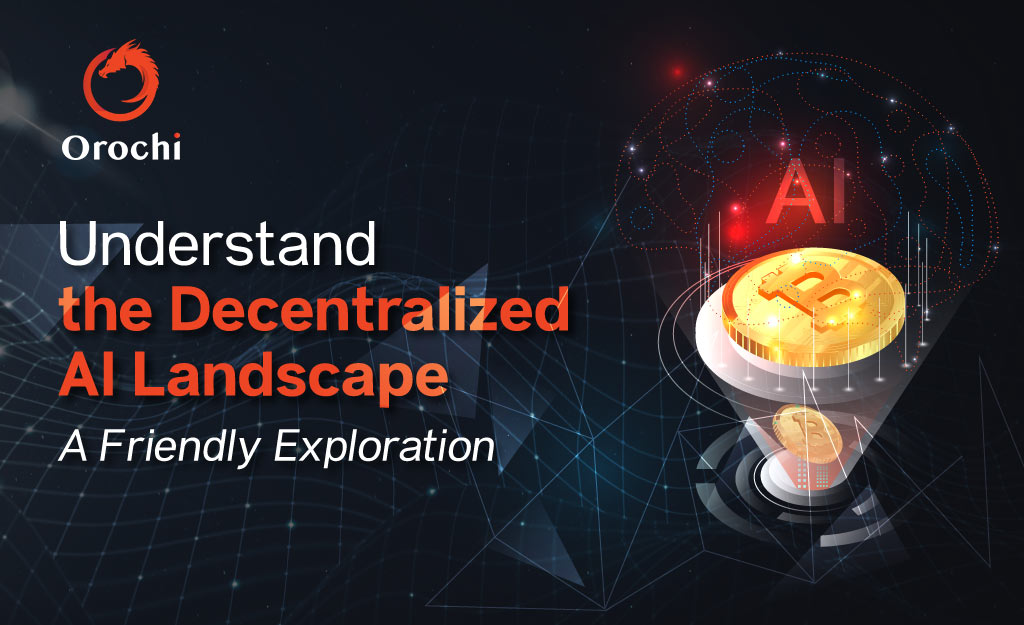
Decentralized AI is emerging as a pivotal theme in the crypto industry. In this article, we embark on a journey to uncover the nuances of Decentralized AI, exploring its potential impact and the projects shaping its landscape.
I. Artificial Intelligence Landscape
Embarking on our exploration of the Decentralized AI landscape requires a closer look at the current state of Artificial Intelligence (AI). In recent years, AI has become the torchbearer of innovation, transcending traditional boundaries and finding applications in a multitude of industries.
Current State of AI
The contemporary AI scene is marked by unprecedented growth and diversification. This surge has been particularly pronounced since the advent of GPT-4 by OpenAI. The capabilities of AI systems have expanded beyond imagination, with builders and developers venturing into uncharted territories. From revolutionizing healthcare through predictive analytics to transforming the creative landscape through generative art and music, AI is a transformative force.
Impact of GPT-4 by OpenAI
GPT-4, the latest offering from OpenAI, has acted as a catalyst for the acceleration of AI applications. Its ability to understand context, generate coherent text, and even engage in meaningful conversations has elevated the standards for natural language processing. As a result, AI projects across the globe are leveraging this powerful tool to push the boundaries of what was once thought possible.
AI Projects Across Industries
The ripple effect of AI's growth extends across a spectrum of industries, each witnessing a paradigm shift in its approach to problem-solving. In the realm of healthcare, AI is aiding in early disease detection and personalized treatment plans. In the arts, it collaborates with human creators to birth entirely new forms of expression. In sports, it analyzes data to optimize performance and strategy. The AI canvas is broad and expanding, promising transformative changes in fields as diverse as finance, education, and beyond.
In this dynamic landscape, the decentralized nature of AI projects introduces a novel dimension. It opens up opportunities for collaborative innovation, ensuring that the benefits of AI are not confined to tech giants but are distributed across a diverse ecosystem of creators, researchers, and users. As we navigate this evolving landscape, it becomes clear that the future of AI is not just about technological advancements but about inclusivity, decentralization, and the democratization of intelligence.
II. Key Components of an AI System
Diving into the intricate workings of an AI system unveils a complex symphony of components, each playing a crucial role in the creation, refinement, and deployment of intelligent algorithms.
Data as Input
At the heart of any AI endeavor lies the raw material — data. Users contribute to this reservoir, providing the system with the necessary information to learn, analyze, and generate insights. This collaborative exchange of data fuels the machine learning engines, allowing them to adapt and evolve.
Algorithms: Design and Functions
Think of algorithms as the architects of the AI world. Meticulously crafted mathematical models, these algorithms are designed for specific tasks, whether it's recognizing patterns, making predictions, or solving complex problems. They form the intellectual backbone, dictating the system's ability to navigate and interpret the data it receives.
Computing Power: The Role of High-End GPUs
Empowering AI systems with the ability to process vast amounts of data requires substantial computing power. Enter high-end Graphics Processing Units (GPUs), exemplified by the formidable NVIDIA H100. These technological powerhouses act as the engine room, executing the intricate computations necessary for machine learning tasks.
Training Process
Training an AI system is akin to teaching it a new skill. The training process involves feeding data into the system, adjusting algorithm parameters, and optimizing machine learning models to enhance performance. This iterative dance between data and algorithms refines the system's ability to make accurate predictions or generate desired outcomes.
Evaluation and Validation
Before an AI system steps into the limelight, it undergoes rigorous evaluation and validation. This phase ensures that the trained model performs as expected and aligns with the desired outcomes. Similar to quality control in manufacturing, this step is essential for maintaining the integrity and reliability of AI applications.
Integration into Applications
For AI to make a tangible impact, it needs to seamlessly integrate into applications and platforms that users interact with daily. This integration step bridges the gap between the intricacies of AI algorithms and the user-friendly interfaces that make these technologies accessible to a broader audience.
Continuous Improvement through User Feedback
The lifecycle of an AI system doesn't end with its deployment; it thrives on continuous improvement. User feedback becomes the catalyst for evolution, guiding developers in tweaking algorithms, refining models, and ensuring that the AI system remains adaptive to the ever-changing landscape of user needs.
As we unravel these components, it becomes evident that the magic of AI lies not just in its algorithms but in the orchestrated synergy of data, computing power, and human collaboration. The journey of building intelligent systems is a dynamic interplay between innovation and user-driven refinement, underscoring the transformative potential of AI in our interconnected world.
III. Computing Power Supremacy
In the realm of Artificial Intelligence (AI), the significance of computing power cannot be overstated. As AI systems continue to evolve and handle increasingly complex tasks, the backbone supporting their operations lies in the supremacy of computing resources, particularly Graphics Processing Units (GPUs).
GPU Resources in AI
GPU resources act as the lifeblood of AI operations, providing the necessary computational muscle to execute intricate algorithms. These processing units are designed to handle parallel computations, a feature that aligns seamlessly with the parallelizable nature of many machine learning tasks. The parallel processing prowess of GPUs enables AI systems to crunch vast datasets and perform complex calculations at remarkable speeds, propelling the capabilities of these systems to new heights.
Challenges for Small Teams
However, with great power comes a significant challenge, especially for smaller teams and independent developers. The cost associated with harnessing the full potential of high-end GPUs can be prohibitive, creating a disparity in access. This financial hurdle poses a real challenge for emerging AI projects, limiting their ability to leverage the full spectrum of computing power required for cutting-edge machine learning tasks.
Investments by Tech Giants (Microsoft, Google, Amazon, Meta)
To address this challenge, major tech conglomerates, including Microsoft, Google, Amazon, and Meta, have recognized the pivotal role of GPU resources in the AI landscape. In response, they have made substantial investments in GPU technologies over the past few years. These strategic investments not only bolster their own AI initiatives but also contribute to creating a more inclusive AI ecosystem.
The infusion of capital into GPU technologies is a testament to the recognition of computing power as a foundational pillar for the future of AI. These tech giants are paving the way for broader accessibility, ensuring that the advantages of advanced computing resources are not confined to the privileged few but are democratized for the benefit of the wider AI community.
As we navigate the landscape of AI computing power, it is clear that the collective efforts of industry leaders are shaping an environment where even smaller teams can tap into the expansive potential of GPUs. This collaborative approach not only fosters innovation but also sets the stage for a more diverse and inclusive AI landscape, where computing power is a shared resource propelling the entire industry forward.
IV. Language Learning Models (LLMs)
Within the intricate tapestry of Artificial Intelligence, Language Learning Models (LLMs) emerge as a pivotal thread, weaving together the intricate fabric of communication, understanding, and innovation. Let's delve into the profound role that these models play in shaping the AI landscape.
Role of LLMs in AI Systems
Language Learning Models are the linguistic virtuosos of the AI world, capable of understanding, interpreting, and generating human-like text. They serve as the bridge between raw data and comprehensible insights, allowing AI systems to navigate the subtleties of human language. Whether it's answering queries, generating creative content, or facilitating seamless communication, LLMs stand at the forefront of natural language processing advancements.
Advanced Models by OpenAI and Google
Pioneering this frontier are industry leaders such as OpenAI and Google, whose advanced LLMs have redefined the benchmarks of linguistic capability. The release of models like GPT-4 and BERT has propelled the field forward, enabling AI systems to contextualize information, understand nuance, and respond with a level of sophistication previously unseen.
Closed Source Nature and Control
However, the journey into the realm of LLMs comes with its own set of considerations. Notably, major players in the AI domain, including OpenAI and Google, have chosen to keep their LLMs closed source. This decision reflects a nuanced approach to control and oversight, allowing these companies to steer the development and usage of their models.
This closed-source nature serves as a double-edged sword. On one hand, it empowers developers to maintain a tight grip on the intricacies of their models, ensuring precision and control. On the other, it sparks debates around accessibility, transparency, and the collaborative spirit that characterizes the broader AI community.
As we navigate the landscape of Language Learning Models, it becomes apparent that these linguistic marvels are not mere tools but integral components shaping the ethical, practical, and collaborative considerations within the AI domain. The ongoing dialogue around openness, control, and responsible AI development underscores the delicate balance required to harness the full potential of LLMs while fostering an inclusive and collaborative AI ecosystem.
V. Building Decentralized AI Systems
In the quest for a more inclusive and collaborative AI landscape, the concept of decentralized AI systems emerges as a beacon of innovation. Breaking away from the traditional centralized models, decentralized AI envisions a distributed and collaborative approach. Let's delve into the foundational elements that contribute to the construction of these transformative systems.
Distributed Computing Resources
The average home GPU, a powerful computational resource, often operates at a fraction of its capacity. Harnessing this latent power, decentralized AI seeks to pool together these underutilized GPU resources, creating a distributed computing network for machine learning. This novel approach not only optimizes existing resources but also democratizes access to computing power, leveling the playing field for smaller contributors.
Empowering Independent AI Researchers
Fueling the engine of decentralized AI are independent AI researchers who, armed with talent and innovative ideas, seek affordable computing resources. Providing these researchers access to the necessary computational firepower is crucial for unlocking their full potential. As they gain entry to these resources, they can contribute to the collaborative ecosystem by open-sourcing their machine learning models, paving the way for continuous improvements and shared advancements.
Validators in the AI System
Validation is a cornerstone of any reliable AI system. Enter Validators – entities that play a critical role in testing trained models before their release to the public. This process bears a resemblance to the consensus mechanisms employed in cryptocurrencies before transactions are added to the blockchain. Validators ensure the integrity and reliability of the AI models, fostering trust within the decentralized AI ecosystem.
The Role of a Decentralized Marketplace
Facilitating connections and value exchanges in a decentralized manner, a decentralized marketplace acts as the marketplace of the future. This platform connects GPU owners, AI researchers, and AI projects, providing a space for collaborative innovation. Transactions within this marketplace are facilitated through crypto tokens, adding a layer of security and efficiency to the value exchange process.
Governance Structure for Decentralized AI
Decentralized AI systems operate on the principles of collective governance. Stakeholders, including GPU providers, AI researchers, projects, and users, collectively contribute to the governance structure. This collaborative approach ensures that decision-making is inclusive, transparent, and aligns with the evolving needs of the decentralized AI ecosystem. Governance becomes the bedrock for continuous improvement and progress within the system.
As we unravel the intricacies of building decentralized AI systems, it's evident that this paradigm shift introduces a new era of collaboration, accessibility, and innovation. From harnessing untapped computing resources to empowering individual contributors, the decentralized approach reshapes the dynamics of AI, promising a more inclusive and equitable future for the field.
Conclusion
As we navigate the landscape of Decentralized AI, projects like SingularityNET have reached a stage of maturity, while others are on the evolutionary path. Investing in these ventures entails a high-risk, high-reward proposition, emphasizing the need for continuous improvement in this competitive space. The decentralized future of AI beckons, promising collaborative advancements and a shared journey towards innovation.
About Orochi Network
Orochi Network is a cutting-edge zkOS (An operating system based on zero-knowledge proof) designed to tackle the challenges of computation limitation, data correctness, and data availability in the Web3 industry. With the well-rounded solutions for Web3 Applications, Orochi Network omits the current performance-related barriers and makes ways for more comprehensive dApps hence, becoming the backbone of Web3's infrastructure landscape.
Categories
Event Recap
3
Misc
56
Monthly Report
1
Oracles
4
Orand
3
Orosign
19
Partnership
20
Verifiable Random Function
9
Web3
102
Zero-Knowledge Proofs
45
Top Posts
1
Introducing Orochi Network - The Operating System For High Performance dApp And Metaverse
10 January 2023
2
Orosign Wallet 101: How to get started?
03 February 2023
3
Validity Proofs vs. Fraud Proofs: An Explanation
06 January 2023
4
Introducing Orosign Multisignature Wallet - A Self-Managing Mobile App For Digital Assets
06 January 2023
5
Introducing X-ORO Points: Opportunity to jump into Orochi Network's Token Whitelist
22 March 2024
6
Discovering the Orochi Retroactive Adventure: Origin, Oro Wild, and Oro Futuristic
21 March 2024
7
Introducing Orand: Your Trustless Source of Randomness
20 February 2023
8
Compete, Connect, Conquer: Orochi Network's Leaderboard Challenge Begins
01 April 2024
Tag
Orand
NFT
Misc
Web3
Partnership Announcement
Layer 2
Event Recap
Immutable Ledger
Oracles
Verifiable Random Function
Zero-Knowledge Proofs
Multisignature Wallet